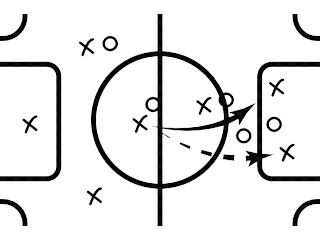
The rise of analytics in sports is akin to how players can compare and choose the best casinos according to Bonus.ca. Just as players seek the best odds and bonuses, sports teams analyze data to optimize their performance. The parallels are striking: both involve sifting through vast amounts of information to make informed decisions that enhance outcomes.
One might wonder—how did we get here?
Historical context: From gut feeling to data-driven decisions
The journey from intuition-based decisions to data-driven strategies in sports has been a fascinating evolution. In the early days, coaches and managers relied heavily on their gut feelings and years of experience. This approach, while sometimes effective, lacked the precision and objectivity that data analytics offers today.
A significant milestone in this evolution was the advent of sabermetrics in baseball. Pioneered by Bill James in the 1970s, sabermetrics introduced a scientific approach to analyzing baseball statistics. This method laid the groundwork for the data-driven strategies we see today.
The technological advancements of the late 20th and early 21st centuries further accelerated this shift. The introduction of computers and sophisticated software allowed for the collection and analysis of vast amounts of data. This technological leap enabled teams to move from basic statistics to more complex metrics that provide deeper insights into player performance and team dynamics.
One of the most notable examples of this shift is the "Moneyball" approach adopted by the Oakland Athletics in the early 2000s. Under the leadership of General Manager Billy Beane, the Athletics used data analytics to build a competitive team on a limited budget. This approach not only revolutionized baseball but also demonstrated the power of data in sports strategy.
Case studies: Success stories in data analytics
Several sports teams have successfully implemented data analytics to enhance their performance. These case studies highlight the transformative impact of data on sports strategy.
Baseball: The Moneyball revolution
The Oakland Athletics' "Moneyball" strategy is perhaps the most famous example of data analytics in sports. By focusing on undervalued players with high on-base percentages, the Athletics managed to build a competitive team despite having one of the lowest payrolls in Major League Baseball. This approach demonstrated that data could be used to identify and capitalize on market inefficiencies.
Basketball: The Houston Rockets
In basketball, the Houston Rockets have been at the forefront of data-driven strategies. Under the leadership of General Manager Daryl Morey, the Rockets embraced analytics to optimize their shot selection. By prioritizing three-pointers and shots near the basket, the Rockets improved their offensive efficiency. This strategy, known as "Moreyball," has influenced the entire NBA, with many teams adopting similar approaches.
Soccer: FC Barcelona
FC Barcelona, one of the most successful soccer clubs in the world, has also embraced data analytics. The club uses data to monitor player performance, optimize training routines, and develop game strategies. By analyzing metrics such as player movement, passing accuracy, and stamina, Barcelona can make informed decisions that enhance their on-field performance.
The tools and technologies behind the data
The implementation of data analytics in sports relies on a range of tools and technologies. These innovations enable teams to collect, analyze, and interpret data effectively.
Wearable technology
Wearable technology, such as GPS trackers and heart rate monitors, plays a crucial role in data collection. These devices provide real-time data on player movements, physical exertion, and overall health. This information helps teams monitor player performance and make data-driven decisions about training and game strategies.
Performance tracking software
Performance tracking software allows teams to analyze data collected from wearable devices and other sources. These platforms provide insights into player performance, team dynamics, and opponent strategies. By leveraging this information, teams can develop more effective game plans and training routines.
Advanced statistical models
Advanced statistical models are used to analyze complex data sets and generate actionable insights. These models can identify patterns and trends that may not be apparent through traditional analysis methods. For example, machine learning algorithms can predict player performance based on historical data, helping teams make informed decisions about player acquisitions and game strategies.